Is your organization harnessing the power of its data? More than ever before, effective data management has transformed from a competitive advantage to a business necessity. Yet many organizations haven’t quite integrated it into their daily operations.
The good news? The democratization of data tools now offers unprecedented opportunities for teams to leverage information for smarter, more strategic decision-making.
According to a 2024 study by McKinsey Digital, by 2030, business data will be “embedded in systems, processes, channels, interactions, and decision points that drive automated actions”—and organizations and teams that aren’t prepared for this shift risk falling even further behind.
In this guide, you’ll discover how implementing a proper data handling plan can improve your organization’s operational efficiency and help secure a thriving future.
Why Business Data Management is That Important
Companies that neglect proper data management can lose valuable insights or fail to deliver data to the right people. Moreover, it can lead to wasting hours searching for information through messy spreadsheets.
With data scattered across the organization, it then gets harder to see the bigger picture and create new opportunities.
However, investing in data strategies enables teams to make better decisions and innovate from it. In that way, it allows the company to preserve its competitive advantage.
Signs That a Company Needs Better Data Handling
Every company deals with data, at one level or another. Thus, it’s important to keep an eye on signs that show the demand for a more efficient management, such as:
- The company’s systems collect huge amounts of data but don’t turn them into knowledge.
- Developing reports has become a complex, laborious, and standardless process.
- The data does not present levels of reliability, requiring several manual checking steps.
- Data comes from too many different systems.
- There are information leaks or problems related to cyberattacks.
Like any strategy, clear principles are key to building a successful roadmap. They guide how to collect, organize, and use data in daily operations. While there’s no one-size-fits-all approach, starting with a clear goal is a good idea.
Business Data: Types and Structures
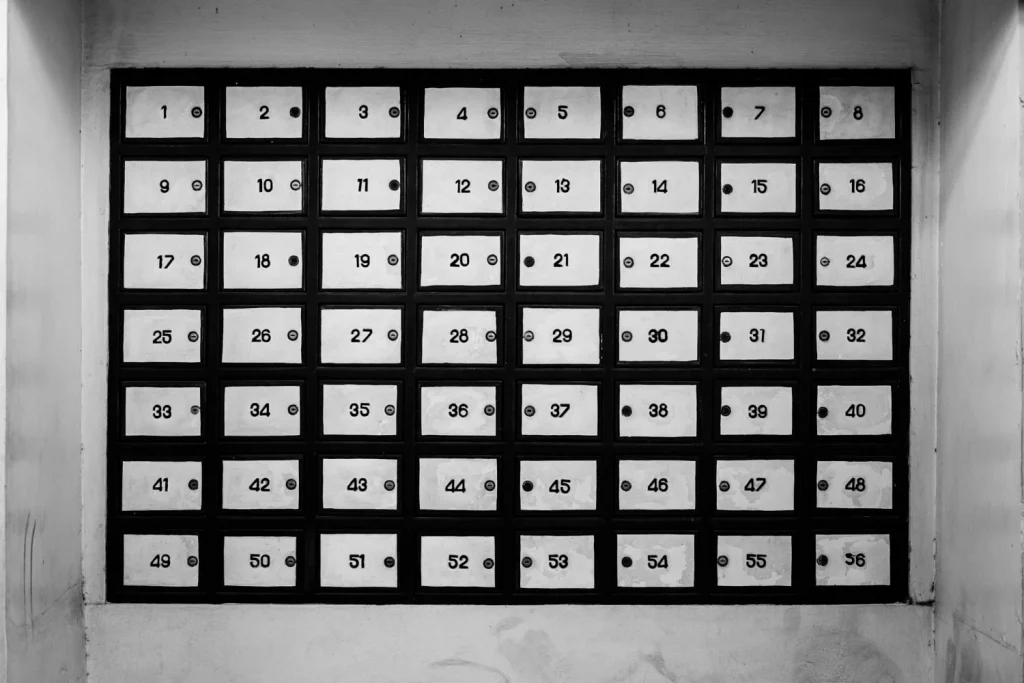
Business data exists in various forms, each with distinct characteristics that determine how to store, process, and analyze it. Understanding these types is essential for implementing productive strategies and deriving observations that move the organization forward.
Let’s explore the three most common ones, beginning with the most structured type:
Data Types (structured, unstructured, and metadata)
Structured data: If you can arrange data into groups based on specific characteristics, then it falls into this category. It’s sorted in rows and columns, making it searchable. For instance, you may have accessed it in a spreadsheet, like Google Sheets or Microsoft Excel, where names, dates, addresses, and logs are there for you to analyze.
Unstructured data: If it lacks organization or structure, it’s unstructured data. Thus, it can be harder to work with compared to structured data. Yet unstructured data can reveal great insights if you know how to organize and analyze it. Examples of unstructured data include images, text, and social media comments and reactions.
Metadata: If it categorizes data and helps ensure compliance with internal and external standards, it’s metadata. It’s controlled by specific rules, like tags and semantic markers.
Understanding different types of data is only part of effective business data management. Now, let’s investigate the most common data storage structures that modern businesses rely on.
Data Storage Structures (or Databases)
Relational Database | Cloud Storage |
Relational databases are structures that store and organize data points with defined relationships between them for quick access. They’re arranged into tables with predefined categories through rows and columns. Structuring data in this way makes access efficient and flexible. | Cloud storage is a digital solution that provides a convenient and cost-effective way to store data. It’s ideal for businesses managing large volumes of information without using up valuable server space. With third-party providers handling maintenance and security, companies can focus on their core operations. |
Data Warehouse | Data Lake |
A data warehouse is a type of data organization system designed to enable advanced business intelligence (BI) activities. It holds large amounts of historical data and derives it from a wide variety of sources, such as application log files and transaction applications. | Unlike a data warehouse, a data lake is a centralized repository for all data, regardless of type. Businesses use them to store raw information without requiring transformation before storage. Through a data lake, applications such as big data analytics and machine learning can access data even if it is completely unstructured. |
Data Management Best Practices
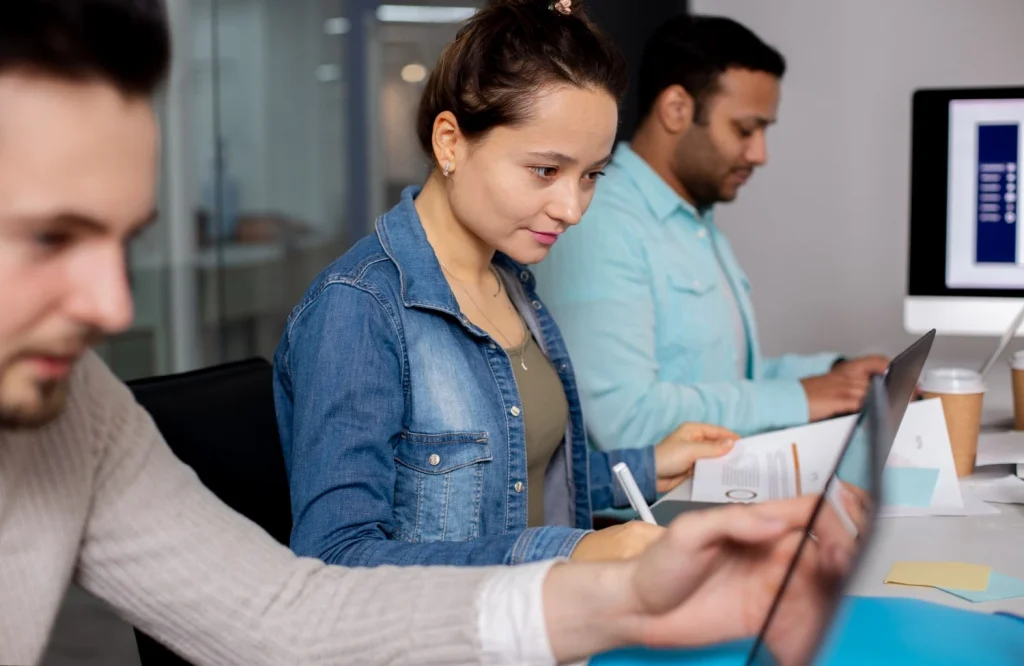
From structuring data to leveraging automation and cloud solutions, following best practices can prevent inefficiencies and data silos. Here are three best practices to ensure a well-structured data environment:
1. Logical Hierarchies, Consistent Naming, and Metadata Usage
Companies experiencing a considerable increase in data volume are standardizing data for seamless access, analysis, and sharing.
But how can you and your team start planning which labels and patterns you will use to classify data? Let’s see:
- Identify key elements that need naming, such as documents, files, folders, and so on.
- Analyze its target audience and usage context.
- Define formatting conventions: Capital letters? Underscores? Which are the permitted characters?
- Keep it consistent throughout the company’s database.
Through the use of metadata, you can add an extra layer that helps you describe data through attributes such as date and time, ownership, responsibility, method, purpose, and even location.
After planning, creating standards, and applying them with the help of data management tools, it’s central to communicate and reinforce them throughout the company.
2. Accessibility and Team Collaboration
Leaders and team members must always collaborate to ensure meeting an organization’s data requirements. All data processing and analysis must focus on business intelligence requirements. Otherwise, the data collected will remain unused, with resources wasted.
3. Automation
We’re prone to making mistakes when handling data. A successful data strategy should incorporate some level of automation into most data processing and preparation tasks, and choosing robust software can support faster and more efficient scalability.
By focusing on these best practices, teams and businesses can avoid common data fragmentation and bottlenecks.
Common Pitfalls in Business Data Management (and How to Avoid Them)
While best practices can improve efficiency, many organizations still struggle with common challenges related to data. Failing to address these common pitfalls can lead to operational inefficiencies, security vulnerabilities, and decision-making blind spots.
Over-Reliance on Spreadsheets
Many businesses still depend on spreadsheets for storing and analyzing data, despite their limitations in scalability, security, and collaboration. While spreadsheets work well for small-scale tasks, they become error-prone and inefficient when handling large datasets.
A more effective approach is adopting unified data platforms that allow real-time updates, access control, and seamless integration across teams.
Lack of Data Governance
Without clear governance policies, businesses risk inconsistent data handling, regulatory non-compliance, and unreliable insights. Organizations that fail to establish role-based access controls, data integrity checks, and compliance frameworks often struggle with data fragmentation and security gaps.
Implementing a structured governance model ensures that data remains accurate, accessible, and secure across departments.
Rigid or overpriced tools
Using inflexible or costly solutions can limit scalability and productivity. Some businesses invest in platforms that lack customization options, while others find themselves locked into expensive plans that don’t align with their evolving needs.
To avoid this, companies should seek scalable, adaptable tools that can grow alongside their data demands without excessive costs.
Poor Access Control & Security Risks
Weak access management exposes businesses to data breaches, unauthorized modifications, and compliance violations. Granular permission settings prevent overexposing sensitive information or restricting it in ways that hinder workflow performance.
Strengthening access control mechanisms, implementing encryption, and auditing user permissions can mitigate these risks.
These common pitfalls create significant challenges for scaling businesses. Recognizing these issues, AnyDB was designed to provide a solution. It provides a centralized platform that replaces scattered spreadsheets and disconnected tools.
Additionally, AnyDB features an intuitive interface that’s as easy as Excel but as powerful as an enterprise solution. This approach helps businesses overcome the data management obstacles that typically hinder growth.
Choosing the Right Tools to Manage Data
A well-structured data handling system should support storage, automation, security, and analytics, helping businesses make informed decisions without workflow constraints.
Here are the main types of data management tools teams can use to streamline processes:
- Data Integration Tools: Platforms that connect and unify data across many sources, ensuring consistency and accessibility for teams.
- Automation Platforms: Tools designed to reduce manual data processing, streamline workflows, and improve accuracy.
- Data Governance & Security Tools: Solutions that enforce access controls, compliance policies, and data integrity standards.
- Data Analytics & Visualization Tools: Software that transforms raw data into intelligence through dashboards and reports.
- Cloud-Based Solutions: Scalable platforms that offer secure storage, accessibility, and processing capabilities without on-premise infrastructure constraints.
While businesses can enjoy using a combination of these tools, finding a unified solution that integrates many features in one platform can further reduce data fragmentation, improve organization, and make monitoring and analysis more seamless.
7 Steps to Implement a Scalable Data Strategy
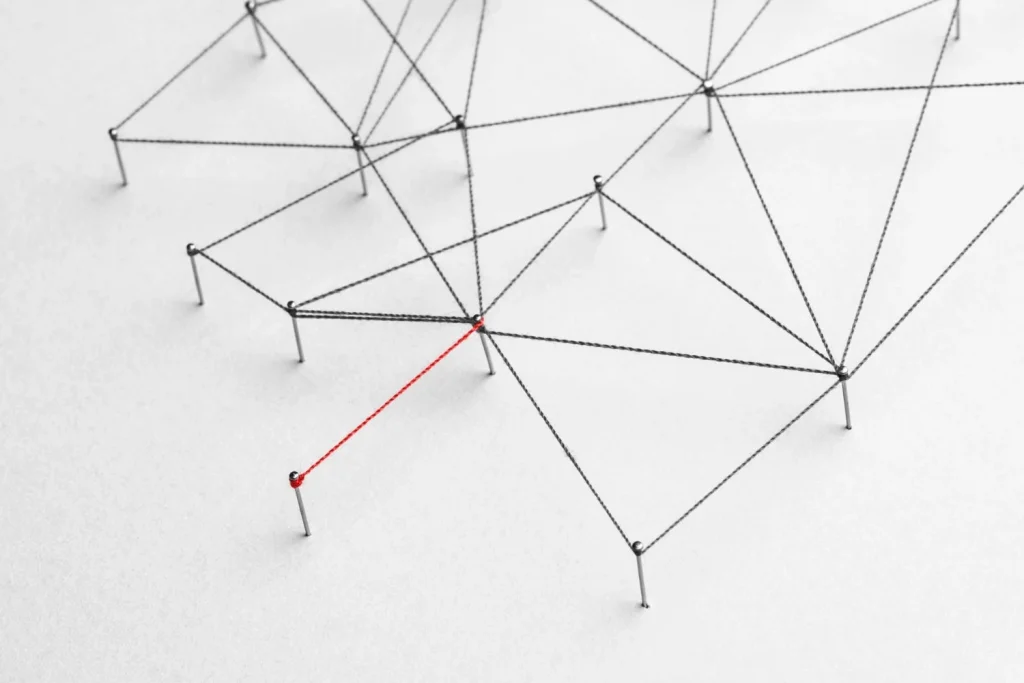
Building an effective data management strategy requires more than selecting the right tools—it involves cultural alignment, structured policies, and continuous optimization. Implementing a scalable approach ensures that data remains accessible, structured, and secure as the business grows.
Below are eight essential steps to establish a successful and adaptable plan:
1. Integrate Data Management into Your Business Culture
A strong data-driven culture starts with recognizing the strategic value of data across all levels of the organization. Embedding it into company policies, objectives, and decision-making processes encourages teams to view data in a new light.
2. Assess Existing Data Structures
Leaders should work together to identify inefficiencies, data silos, and security gaps in current practices. Leaders should work together to identify inefficiencies, data silos, and security gaps. This assessment helps define the core priorities of the new strategy.
3. Define Roles and Responsibilities
A well-structured strategy needs clear ownership. Assign dedicated data stewards to oversee implementation, security, and policy compliance. Even when using automation tools or outsourcing certain tasks, having internal accountability is vital for long-term success.
4. Develop a Comprehensive Plan
After ownership alignment, the next step is to define a clear roadmap that includes:
- Goals: What the organization aims to achieve with better data control.
- Resources: The budget, tools, and personnel required.
- Processes: Data collection, storage, integration, and governance strategies.
- Timelines: A phased approach for implementation and optimization.
This approach ensures strategies align with business goals and remain adaptable to future needs.
5. Select the Right Tools
Choosing a flexible platform or set of tools over an outdated system is decisive to centralizing, controlling, and scaling data.
6. Foster Cross-Departmental Collaboration
Although IT teams play a major part, data administration is a company-wide responsibility. Firms looking to minimize inconsistencies and errors need to provide training and support across all teams.
7. Track, Optimize, and Scale
Managing data requires regular monitoring and adaptation. Businesses should:
- Define key performance metrics to track data.
- Audit security measures to prevent breaches.
- Optimize workflows based on team feedback.
- Scale solutions as data needs evolve.
Businesses focusing on continuous improvement can ensure their management strategies remain fertile.
Building a Data-Driven Business for Long-Term Success
Now, it’s time to put these strategies into practice. Armed with this guide, you can kickstart or enhance your data management initiatives.
A final friendly word of advice: Keep reading the AnyDB blog for more on the power of well-managed data.